Apheris: solving the data privacy dilemma
By James Nurton, freelance writer
How do you enable sophisticated artificial intelligence (AI) tools while respecting the privacy and protecting the intellectual property of data assets? A Berlin-based startup believes federated learning provides the answer.
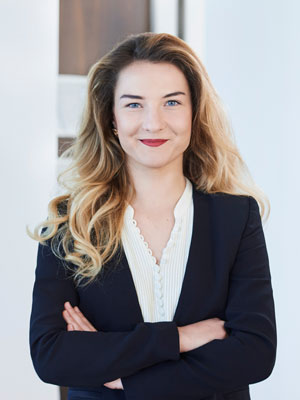
Federated learning is based on the belief
that “sensitive data is best kept local and
under the control of the data controller,”
and delivers results that are “as good as
if you had all the data on your own servers,”
says Lucie Arntz, Head of Legal at Apheris.
(Photo: Courtesy of Apheris)
In his opening speech at the fourth session of the WIPO Conversation on IP and Frontier Technologies in September 2021 (read Data: the fuel transforming the global economy), WIPO Director General Daren Tang described data as the “fuel” that powers digitalization. Algorithms for machine learning require large volumes of data to learn from – but what happens when the flow of fuel is interrupted, in other words when the data cannot be shared for reasons of privacy, security or intellectual property (IP) protection?
One solution to that problem is known as federated learning, where the data never leaves the control of the data owner. Rather, the machine learning algorithms are trained on the data locally, without it ever being shared. In a simple example, sensitive data such as patient records from a hospital can be used in the development of a new drug by a pharmaceutical company without the hospital having to disclose any data. In more sophisticated cases, data from multiple sources can be used to train the same algorithm, bringing benefits in both volume and diversity.
Federated learning requires a trusted third party to bring together the algorithm and data owners. Berlin-based startup Apheris, which was launched in 2019, is one such company. Apheris has a team of about 20 developers, privacy experts and data scientists who provide a secure platform for secure data sharing. Its Head of Legal, Lucie Arntz, recently spoke to the WIPO Magazine about the company’s business model, data protection and security.
Benefits of federated learning
Ms. Arntz joined Apheris in summer 2020 – the first employee not to be a scientist – and is responsible for ensuring a proper legal foundation, protecting customers’ rights and overseeing contracts. She says that federated learning is based on the belief that “sensitive data is best kept local and under the control of the data controller” and that it delivers results that are “just as good as if you had all the data on your own servers”.
Up to now, the benefits have been most evident in the healthcare sector, where AI techniques are advanced and there are fundamental concerns about confidential and sensitive patient data. But Ms. Arntz points out that federated learning offers benefits even where data is not sensitive with respect to personally identifiable information (PII). For example, Apheris is now working on a project for a chemicals manufacturer, which involves product and customer data that is commercially sensitive and secret. Federated learning could also apply where certain data is protected by IP rights.
“Centralizing data is becoming outdated,” says Ms. Arntz, who adds that many companies own large amounts of valuable data which is not leveraged because of concerns about sharing: “You might have lots of data that could be super important to someone else but not to you, so without partnering with someone there’s no value in that data at all.”
In some cases, the value of data might only be apparent when it is combined with data from other sources through federated learning. For example, medical data from patients in the United States could be supplemented with that from Africa or Asia, resulting in a more diverse clinical trials dataset. “You could scale it up as much as you wanted and that’s where it gets magical,” says Ms. Arntz.
But she adds that the potential of federated learning is still probably three years away from being fulfilled. One reason is the need for more standardization in the collection and formatting of data. While increased computing capacity enables the processing of larger volumes of data, for optimal results that data needs to be well structured to enable secure data collaborations. Here, again, the healthcare sector is leading the way, but other sectors are catching up. One that Ms. Arntz identifies is the automotive industry, where the development of partially and fully autonomous vehicles depends on analysis of a great variety of data from various sources – including drivers, vehicles, highway authorities, law enforcement agencies and insurers. “The automotive industry is very focused on getting that standardization in place,” she says. “There’s great interest in being able to collaborate on that data and there are efforts to get the big manufacturers together to standardize. It’s a particularly interesting area because it involves both public and private sector interaction.” In the automotive sector, the solution is likely to be voluntary and industry-led, but it will take time to develop.
While increased computing capacity enables the processing of larger volumes of data, for optimal results that data needs to be well structured to enable secure data collaborations.
The anonymization conundrum
One big challenge for the development of AI tools is the level of anonymization. Individuals are understandably concerned to protect their personal data (whether medical or family history, financial information or other personal details) but, as Ms. Arntz says, “the more anonymized the data, the less relevant it becomes. Anonymization is not the future of machine learning.” Effective drug development and testing, for example, needs to take account of age, ethnicity, allergies, medication and other factors; self-driving cars need information on where you’re travelling to, what kind of vehicle you drive and how fast you want to go. Ms. Arntz believes federated learning can help provide a balance and show that “it’s not a conflict to have both privacy and innovation.”
Overcoming such challenges requires a mix of technological and legal solutions: the technology can ensure the security of data through processes that are rigorous and intensely tested, while law enables contracts that stipulate who controls the data, who can receive the results and what level of detail they receive.
Comparing Centralized and Federated Learning

"Centralizing data is becoming outdated," says Ms. Arntz. “You might have lots of data that could be super important to someone else but not to you, so without partnering with someone there’s no value in that data at all.”
How data is actually protected remains a difficult question: while copyright law and sui generis tools such as database rights in the EU might offer some protection, the boundaries are not clear and most organizations are likely to favor keeping data secure, relying on contractual provisions and protection under trade secrets or confidential information laws. But Ms. Arntz says the question of whether and how data is protected need not be a problem: “If you have data, you probably think it’s important and should be protected. For federated learning, it does not matter whether the data is protected formally or not. We err on the safe side.”
A more pressing issue, she believes, is “broad consent.” The GDPR recognizes that it is not always possible for scientific researchers to identify all the purposes for which data is collected. Therefore, they may not have to be as specific about their plans in other areas, but should, nevertheless provide options so that data subjects can give informed consent for future research uses. “We need clearer guidance on what “research purposes” are. At the moment, there is uncertainty for universities and researchers and that is limiting innovation,” she says.
Shining a light on fair regulation
Ms. Arntz believes the GDPR is an example of legislation that is “much-criticized but also much loved”: it provides a sound basis for data protection but will need to be updated as technology changes. “Above all, we need clarity: even if the guidance is that you can’t do something, at least it’s good to have a clear line.”
She also argues that the GDPR is an example of how a region – in this case, the EU – can “shine a light” to promote fair regulation: as she says, data cannot merely be regulated nationally so multinational or international solutions are needed – even if compromises have to be struck along the way. She is optimistic that new EU initiatives, such as the recently adopted Data Governance Act and the proposed AI Act, will provide further clarity: “Policy should be always open to optimize. We will need to adapt it in future and revisit what we’re trying to achieve.”
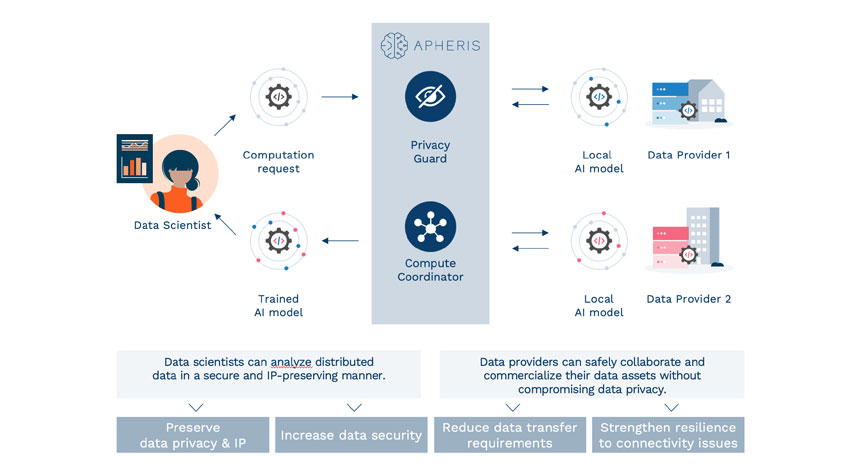
Apheris enables companies to securely analyze data of multiple parties while keeping proprietary information private.
She warns though, that the process must be inclusive and interdisciplinary: too often the business, legal, policy and technical experts are not in the same room or even talking the same language, and the voice of startups and SMEs is not always heard. “Governments talk to big corporations a lot but if they’re not talking to startups so they don’t hear about innovative technology,” Ms. Arntz explains.
The conversation is important, she says, because the technology is getting more and more sophisticated, and there is abundant funding available for new products and services that are derived from AI and data analysis. The importance of data is apparent in everything from tackling the COVID-19 pandemic to assessing the impact of climate change. “We’re going to see lots of growth in data analysis, and the policy will have to move in response,” says Ms. Arntz.
Data in the EU – existing and proposed
General Data Protection Regulation (GDPR): The 2016 GDPR superseded the EU Data Protection Directive and regulates the processing of personal data of data subjects in the European Economic Area. It has been followed in many other countries and regions, for example in the California Consumer Privacy Act (2018).
Data Governance Act: The Act was adopted by the European Parliament on April 6, 2022. It is heralded by the European Parliament as a move that “will stimulate innovation and help startups and businesses use big data.” The rules will benefit business by lowering the cost of data and market entry barriers. Consumers will benefit, for example, by having access to smarter energy consumption and lower emissions. The rules are also designed to build trust by making it easier and safer to share data by ensuring it conforms with data protection legislation. They will also facilitate the re-use of certain categories of public sector data, increase trust in data intermediaries and promote data altruism (the sharing of data for the benefit of society). The Act will create “the processes and structures” to make it easier for companies, individuals and the public sector to share data. It will have to be adopted by all EU countries in the Council before it becomes law.
EU Data Act: The Act, also known as the Proposed Regulation on Harmonised Rules on Fair Access to and Use of Data, was adopted by the European Commission in February 2022, and is a key pillar of the European data strategy. It clarifies who can create value from data and the conditions under which they may do so.
Artificial Intelligence Act: The proposal for an AI Regulation to lay down harmonized rules for the EU is part of the European Commission’s AI package published in April 2021. It is the first attempt to “enact a horizontal regulation of AI,” and is designed to turn Europe into the global hub for human-centric and trustworthy AI.
The WIPO Magazine is intended to help broaden public understanding of intellectual property and of WIPO’s work, and is not an official document of WIPO. The designations employed and the presentation of material throughout this publication do not imply the expression of any opinion whatsoever on the part of WIPO concerning the legal status of any country, territory or area or of its authorities, or concerning the delimitation of its frontiers or boundaries. This publication is not intended to reflect the views of the Member States or the WIPO Secretariat. The mention of specific companies or products of manufacturers does not imply that they are endorsed or recommended by WIPO in preference to others of a similar nature that are not mentioned.